Antibody Engineering
Accelerating antibody engineering with in silico solutions
Context
Generative AI powered in silico antibody engineering
Computational methods allow us to search and design antibodies effectively in a highly expanded space by combining Generative AI, Denoising Diffusion Probabilistic Models (DDPM) and Machine Learning models of downstream properties.Our proprietary algorithms and computational pipeline seamlessly integrate sequence and structure based design considerations, and incorporate multiple property criteria enabling the design of antibodies with improved antigen binding and developability.
Our Solution
Powered by Generative AI
We leverage cutting edge computational technologies including Generative AI, Denoising Diffusion Probabilistic Models and Machine Learning for therapeutic antibody engineering.
Our algorithms combine antigen binding affinity, specificity, and stability to produce desired candidate antibodies. Seamlessly integrating sequence, structure, and multiple property criteria, our proprietary pipeline enhances antibody design for improved antigen binding and developability.
Modular, Customizable Pipeline and AI models
Our modular technology can adapt to any antibody discovery stage- starting from disease, antigen, hit, or lead with liabilities. Our experts design antibodies with desired properties from concept to optimization
End-to-end support
We offer comprehensive project support, including customized pipeline development, in vitro antibody validation, and publication assistance
Align antibody design for clinical success
Mitigate candidate risk by assessing manufacturability, immunogenicity, potency, and humanness using our in silico developability toolbox
Accelerated antibody therapeutic development
Our in silico pipeline rapidly generates leads with a higher chance of success in the downstream stages of in vitro, in vivo and clinical testing
Highlights
Key components & strengths
Sequence and structure generative models
Our generative AI models jointly model sequence and structure, and developability properties, to generate antibodies with strong binding and developability characteristics.
Innovative deep learning and equivariant neural network model
Our models leverage denoising diffusion probabilistic models (DDPM) to broaden the search space of candidate antibodies and address the inherent challenge of antibody property data insufficiency
Multiple antibody formats
Our models span monoclonal, single domain, bispecific, and multispecific antibodies
Customized pipelines
We are able to customize and fine tune our approach and optimize our in silico toolkit to meet the specific needs of each project, whether it is hit identification, hit to lead optimization or developability property optimization
Virtual screening of large antibody libraries
Our technology supports virtual screening of antibodies, analysis of antigen-antibody complex structures, and progressing to final optimized antibody candidates, taking into consideration multiple aspects related to their developability
Our Antibody Engineering offerings
Antibody Virtual screening
In silico pipeline to evaluate a large library of antibody candidates and produce rank order list of hits based on binding affinity and developability properties.
Read More
Antibody Lead Optimization
Generative AI based model to optimize properties such as humanization, aggregation optimization, stability improvement, etc. for increased precision.
Read More
Antibody Characterization
In silico developability property profile of the antibody including thermal stability, isoelectric point identification, hydrophobic patches, aggregation propensity, humanization score, etc.
Read More
Sequence Structure Co-Modeling
Explore the unexplored designable antibody space with generative AI models while considering sequence and structural co-modeling to design antibodies for a target antigen of interest.
Read More
Sample Use Cases
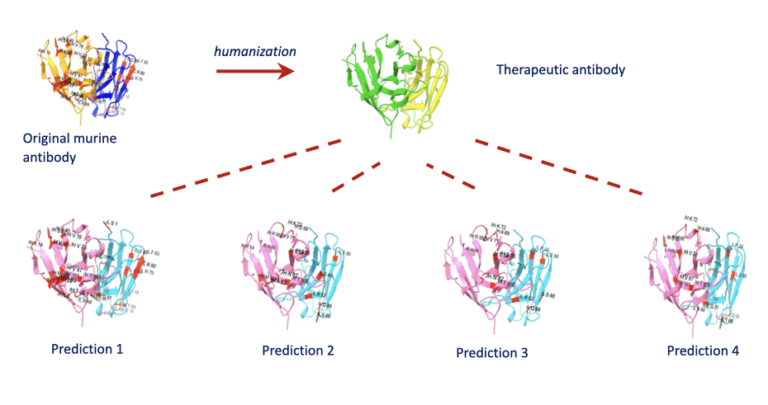
Humanization
Deep learning based methods show the best performance in humanizing antibodies in silico
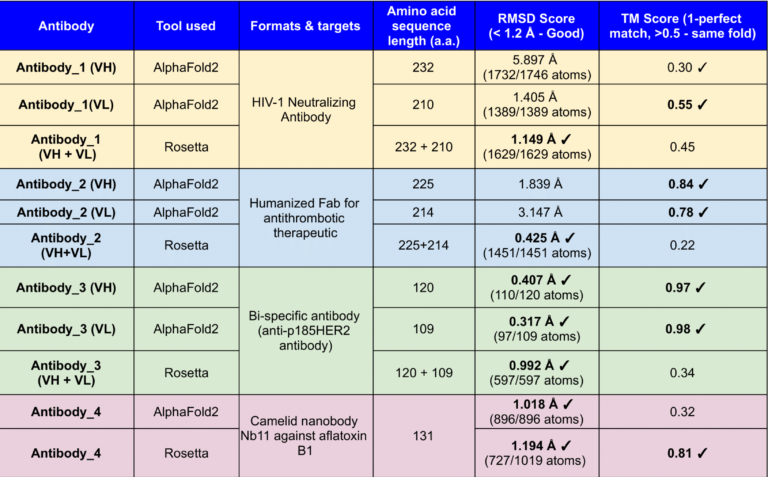
Structure Prediction
RosettaSuite outperformance AlphaFold2 when considering antibodies
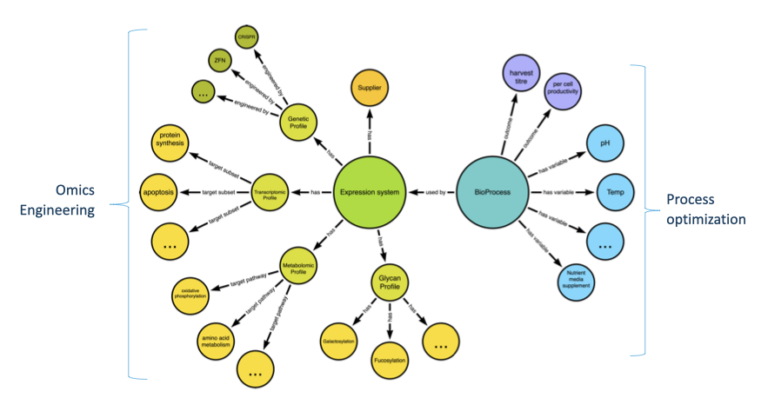
Yield Optimization
Both Omics and BioProcess domains can contribute to decision making
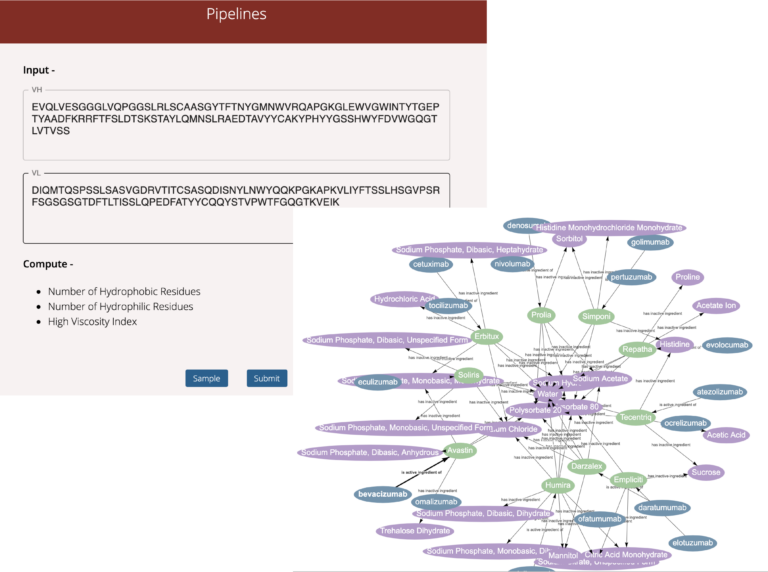
Stability Estimates
Details of therapeutic mAbs and pipelines to estimate hydrophobicity and viscosity
Outcomes
Accelerate Antibody Therapeutics: R&D Enhanced by in silico Solutions
Revolutionize antibody design through structure guidance
Unlock previously unexplored antibody sequences using our state of the art generative AI and structure-based discovery process
Maximize antibody affinity
Predict higher affinity antibodies through affinity maturation, unlocking their full therapeutic potential
Achieve precision target recognition
Accurately predict antibody developability, humanness, and affinity to target antigens
Closed loop with in vitro testing
Combine in silico and experimental testing (in vitro, in vivo) to accelerate antibody research while reducing experimental expenditure
Discover our offerings across the biopharma value chain
Our Solutions
Customizable platforms and solutions across the drug discovery value chain from target discovery to therapeutic development
Read More
Our Services
Offering services in computational sciences and technology to complement biopharma R&D
Read More
Case Studies
Helping clients accelerate drug discovery and development by building computational biology, computational chemistry, technology, and cloud solutions
Read More